Abstract
The efficient exploration of chemical space to design molecules with intended properties enables the accelerated discovery of drugs, materials, and catalysts, and is one of the most important outstanding challenges in chemistry. Encouraged by the recent surge in computer power and artificial intelligence development, many algorithms have been developed to tackle this problem. However, despite the emergence of many new approaches in recent years, comparatively little progress has been made in developing realistic benchmarks that reflect the complexity of molecular design for real-world applications. In this work, we develop a set of practical benchmark tasks relying on physical simulation of molecular systems mimicking real-life molecular design problems for materials, drugs, and chemical reactions.Additionally, we demonstrate the utility and ease of use of our new benchmark set by demonstrating how to compare the performance of several well-established families of algorithms. Surprisingly, we find that model performance can strongly depend on the benchmark domain. We believe that our benchmark suite will help move the field towards more realistic molecular design benchmarks, and move the development of inverse molecular design algorithms closer to designing molecules that solve existing problems in both academia and industry alike.
Introduction
Inverse molecular design, which involves crafting molecules with specific optimal properties [1],poses a critical optimization challenge prevalent across various scientific fields. It holds particular significance in chemistry for designing drugs, catalysts, and materials. Each of these scenarios requires a complex balance between multiple desired properties for a realistic inverse design. Yet, the majority of comparisons between molecular design algorithms are primarily carried out on oversimplified tasks, thereby providing limited insights into their potential performance when confronting intricate design challenges commonplace in chemistry [2–4]. While simplistic and cost-effective benchmarks serve as vital tools during the initial stages of algorithm development, facilitating rapid testing and informed design choices, the lack of more realistic benchmarks obstructs their adoption by domain experts in chemistry or materials science. Our work aims to bridge this gap.In this study, we propose a modular suite of design objectives directly inspired by problems regarding new materials, drugs, and chemical reactions. These problems rely on well-established simulation methods in computational chemistry, such as force fields, semi-empirical quantum chemistry, and density functional approximations. We test multiple popular molecular design algorithms on these objectives, offering an exemplary performance comparison. The design approaches selected represent some of the most important algorithm classes in the field. We also examine the impact of representations on the performance of some algorithms. Finally, we provide detailed instructions for installation and setup for both the benchmarks and the molecular design algorithms, enabling the cheminformatics and machine learning communities to integrate them into their work and inspire the development of future methods. The core idea is to provide a unified benchmarking framework with a diverse selection of problem domains to assess the generalizability of inverse design algorithms.Notably, the scope of included benchmark domains is to be expanded upon in future work. Altogether, we believe this is a stepping stone towards the widespread adoption of molecular generative models for challenging design problems that permeate the chemical sciences.
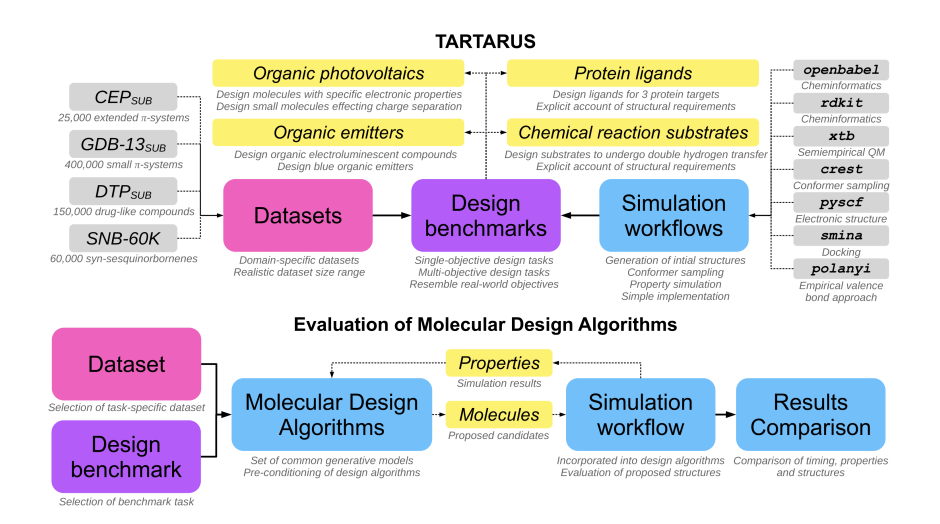
Figure 1: TARTARUS framework, highlighting its two core elements. Top: Real-world design tasks are defined and paired with simulation workflows and datasets. Bottom: The Evaluation Pipeline:Generative models are conditioned using reference datasets, sampled for structures, and evaluated based on their properties through custom simulation workflows. Model performance is assessed based on the alignment of the acquired properties with predefined objectives.